Remember when Friday night meant a family trip to Blockbuster, browsing aisles of VHS and DVD titles, hoping the popular new release was still in stock? At its peak, Blockbuster boasted over 9,000 stores worldwide and nearly $6 billion in revenue, dominating home video and game retail. Seemingly overnight, Blockbuster went from a family Friday staple to a tailspin, closing stores across the globe and filing for bankruptcy in 2010.
What led to Blockbuster’s downfall? How does it relate to AI? We’ll explore those two questions in this blog.
What led to Blockbuster’s downfall?
As we know now, consumers would shift from DVD rental in-store to online streaming video as the primary means of consumption. While this is easy to see now, in the early 2000s there were a lot of barriers. Streaming technology was choppy and not connected directly to your TV, and the content licensing model for streaming was unclear.
Sometimes when we look back at this transition, we think that Blockbuster was completely asleep at the wheel, blissfully unaware of the digital transition. But this wasn’t actually the case, the exhibit below is from their 2005 financial statement, about 2 years before Netflix launched digital.
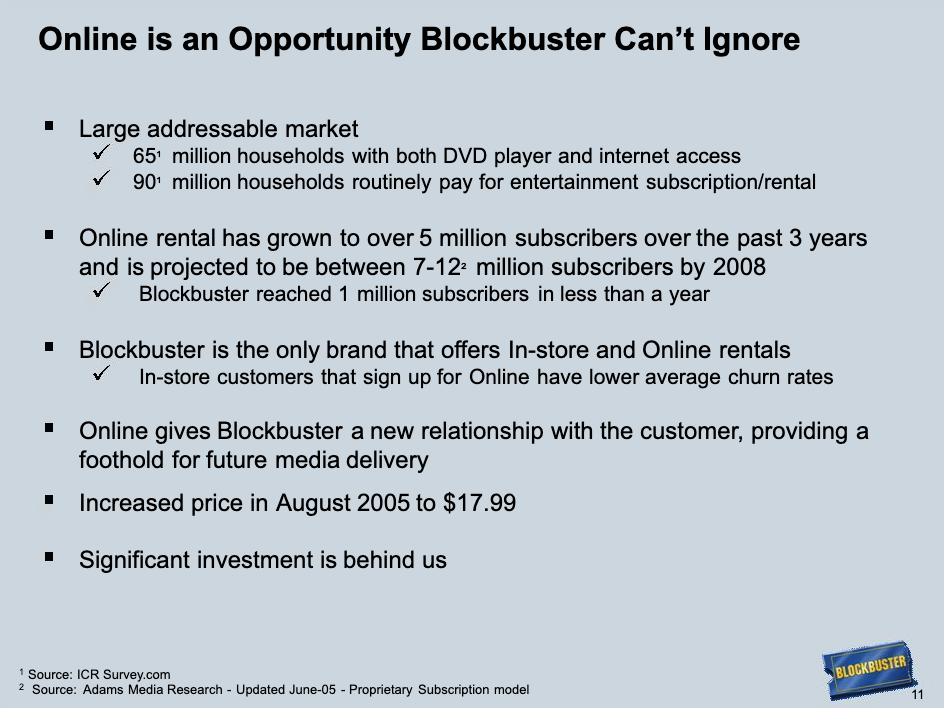
What led to Blockbuster’s demise was not the lack of awareness in the transition to digital, but the lack of a future vision for streaming and process to create an iterative roadmap to get there. In dynamic transitions, the iterative process of understanding and updating is critical to get past false peaks like online ordering of DVDs as the “digital transition”.
Where are we in the AI adoption cycle?
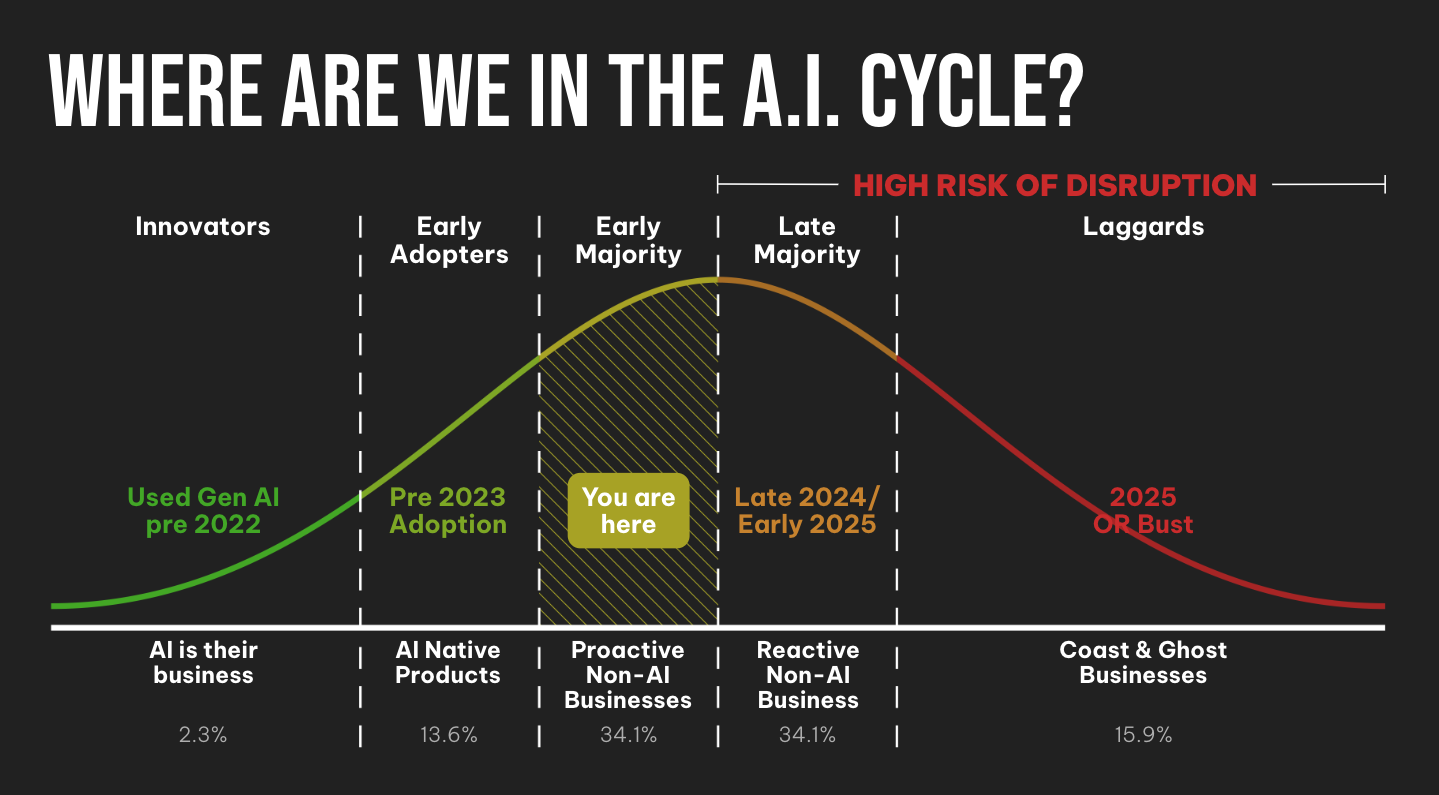
Based on indicators we see in adoption surveys, we are squarely in the early majority phase of adoption with between 15-30% (depending on industry) of people using AI almost every day in their work life. History shows us that tech adoption cycles are accelerating in their overall pace, given the historical trend and the high level of investment across both the VC and big tech players. We expect AI to move VERY rapidly through the adoption curve.
How can you avoid your Blockbuster outcome?
As a product leader, I’ve never thought of my role as one of being an “idea genius” or even a “visionary”, creating great products repeatedly, and at scale involves a little creative magic, but it mainly relies on creating a repeatable and always-on process that turns market and customer feedback into a prioritized set of initiatives. I think success in AI will be a similar recipe and we’ve built the SOVA AI Transformation offering to establish 4 phases of continuous improvement. It’s important to note that these should be continuous, as AI adoption is neither a singular event nor a use case.
Research
Research is about knowing three things: AI technology, your ecosystem, and your company. The best use cases of AI (and all technology really) take existing business opportunities that are now more feasible or can be executed better based on the emerging technologies. During the research process, we marry focused AI training, discovery workshops, and stakeholder and consumer interviews to create a clear picture of today’s challenges and opportunities. This enables us to create a company AI vision and strategy: a key component that was missing for Blockbuster.
Planning
Once you know where you are going, it becomes much easier to figure out how to get there. Our planning phase helps prioritize potential initiatives to develop a company AI roadmap and digs into the top priorities to create an executable plan. This involves conducting a build/buy analysis as well as a deeper dive on ROI and key metrics to monitor. KPI and Metric monitoring can often be time-consuming, but creating a small number of leading indicators and key results is critical to making sure that both your initiatives and the ecosystem continue to evolve as you expected.
Development
Despite the introduction of AI technologies, you don’t need to reinvent the wheel on developing solutions, but there are a few key areas you should be aware of.
- Build/Buy - You may have noticed, there are a TON of new AI tools and technologies coming to market. Because of the relative immaturity, many of these are useful, but partial solutions. There may be more opportunities than in most scenarios for custom tools and creating a customized - multi-tool system.
- New Technologies - Given that most AI forms are available as consumption-based API’s there isn’t a steep learning curve to start adopting, but there IS a huge efficiency gain in knowing the unique challenges, pitfalls, and best practices for dealing with the variable responses for AI systems.
We help clients navigate both these challenges, as well as gain efficiency from our library of reusable AI application components.
Rollout
It’s important to remember that AI is still new in most people’s daily lives. Because of this internal consumers or customers have a wide range of expectations and fears around adopting AI. It’s critical for success to set expectations on performance and usage before your AI tools roll out broadly. This can avoid stalled adoption and in some cases damage to your brand with the wrong product positioning.
Conclusions - Getting it right
Understanding that AI is going to change things is the easy part, creating and executing a winning AI strategy is hard, but for those companies that invest, it will catapult them to being the industry leaders of tomorrow. It’s important to remember that there isn’t a silver bullet - but there is a golden compass, and the AI transformation process can help you navigate and push forward innovating and executing your strategy as your competitors hit roadblocks and uncertainty.
Ready to take your business to new heights with AI-driven solutions?
Start here. Go anywhere.